WHITE PAPER
Health Care Analytics: Enabling the Performance Improvement Conversation
When the Advisory Board Company recently surveyed 146 C-suite executives from health care organizations (HCOs) in the United States, two-thirds of the respondents said their top priorities were reducing costs and preparing their organizations for sustainable cost controls. They believe these issues can’t wait to be addressed. Action is necessary now, today, not two to five years from now when those emerging technologies begin to deliver on anticipated value propositions in health care.
While the need for cost containment is clear, how to achieve it is complex and must consider factors such as practice variability, unnecessary utilization, inefficient resource utilization, and provider productivity. The key to accomplishing these important initiatives is analytics. Analytics can help identify opportunities for improvement and reinforce behaviors through the performance improvement conversation. To do this, a holistic, accessible, and trusted view of all relevant data is required…
Trusted by Your Peers
We transform data for dozens of leading hospitals and health care organizations across the U.S.
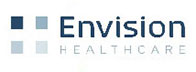
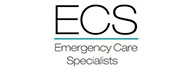

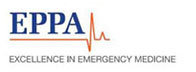

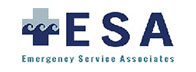
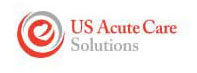
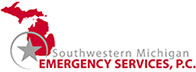


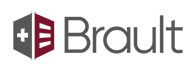

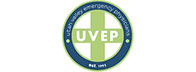




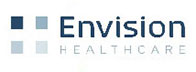
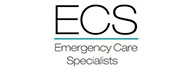

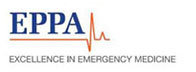

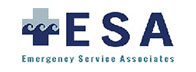
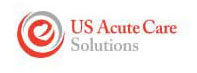
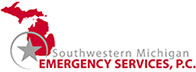


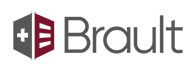

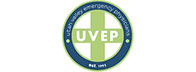



