WHITE PAPER
Armed With Data, Physicians Are Poised to Lead Health Care Transformation
Summary
With purpose-built analytics, health care organizations (HCOs) can pursue dynamic, productive, and high-impact performance improvement conversations, driving action and creating value. The best path forward is one where physicians lead a data-driven consensus-building process that optimizes access and outcomes while reducing costs.
Positive change is promoted when physician leaders — those who actually have the power, mission, and right interests in mind — are given the ability to lead with trustworthy, contextually relevant data. If an organization or department really wants to adapt and evolve, it needs to give physicians the tools they need to lead. So far, this has been slow to happen.
In health care, fewer than 50% of CIOs trust their data, according to a 2019 Kaufman Hall survey on performance management trends and priorities. The lack of clean, consistent, and trusted data is a big barrier when it comes to credibly translating value-based care strategy to frontline execution.
Lack of trust in data puts unnecessary stress on providers who already are under pressure having to spend too much time interacting with their EHR system, and reviewing too many metrics based on data that isn’t always relevant and doesn’t always add up. To improve trust, data management is key, and clinical leaders are vital to implementing data governance and data quality programs to not only promote data integrity, but also to ensure that it’s relevant and actionable.
As we shift toward value-based care, emergency departments (EDs) and hospitals are primary targets for cost reduction. One area under great scrutiny is unscheduled care, which comprises 30% of the 1.2 billion outpatient visits each year in the United States, including 150 million ED visits. In this segment, hospitals have seen declines in revenue and profitability, as well as closures. And now that COVID-19 has laid bare the many cracks in our health care system, the impetus for change will increase.
A decline in inpatient volumes and revenue is a new reality, necessitating a new paradigm, one where:
- More than 50% of health-system revenue comes from ambulatory services and telemedicine.
- Patients who may previously have been admitted instead are safely discharged and referred to in-network services.
- Emergency medicine takes on more responsibility for reducing readmissions and returns.
- Low acuity ED visits are guided to appropriate, lower-cost health care.
Proving Value With Data
For practices to thrive — and to minimize provider burnout — leadership must come from physicians as necessary change agents. Payers, administrators, and patients should look to them for guidance on the changes needed to make health care more cost-effective.
In this paper, we will use emergency medicine (EM) as one health care specialty to describe the value of and process for providing trusted, relevant, and actionable data. EM is where d2i has had the most experience, but the core principles described here apply to all specialties.
In the future, the solution is not to de-emphasize EM, but rather to re-engineer how unscheduled care is managed, enhancing value.
This new paradigm emphasizes safely discharging patients who might otherwise be admitted, improving care transitions, and leveraging telehealth. We expect the COVID-19 pandemic to accelerate movement toward this new normal. Better data is needed to plan, re-engineer, and support a paradigm that emphasizes the quality of output versus the quantity of throughput.

The Challenges of Data-Driven Performance Improvement
HCOs need physician leaders to act as change agents who enable the organizational discussions and collaboration necessary to drive change, and ultimately, value. Access to timely, trusted, and actionable data allows physicians and their teams to monitor progress at all levels of detail, as well as causes and effects from all perspectives: clinical, operational, financial, regulatory, and patient experience.
Providing such a solution requires an intensely focused process that yields datasets that can satisfy even skeptical physicians with data that is trusted, contextually relevant, and easily provides answers to all of their questions.
Defining the Data Value Chain
The data value chain is a process where data in a raw state is taken from multiple sources and leveraged for greatest value. At various stages of the process, the value of the data increases in terms of:
- Its integrity
- Its fitness for specific purposes
- The range of analytic use cases that are supported
- The ease with which new analytics may be created without special programming and new data asks
- How often the data is used
- How it enables quality and process improvement
The picture below illustrates the various stages. The value of the data increases as you advance through the chain, from left to right. We will describe each stage in detail.

Planning: The role of physician leaders is critical during the planning stage, when key decisions are being made about goals, use cases, data rules, and resources. Physicians — through their training, mindset, and role — are uniquely positioned to rise above narrow interests to determine data-driven strategies to balance cost, access, and quality.
While physicians must lead, they generally don’t have the time or experience to build a data management infrastructure to support their requirements. In the planning phase, the following questions should be considered:
- What are we trying to accomplish and what are the desired outcomes?
- What are the key performance indicators (KPIs) that will show progress toward these outcomes?
- What variations in clinical adherence and operations drive KPIs?
- What data is needed to support what we are trying to accomplish?
- Are we ready with the necessary:
- Infrastructure?
- Resources?
- Skills?
- Processes?
- Incentives?
- Have we standardized how metrics are calculated, KPIs are tracked, visits are attributed to providers, and patients are dispositioned?
- What methods are best for delivering performance data to clinicians?
By addressing these and other business questions, physicians fulfill a vital role in data governance, data quality, and ultimately in the value derived from the data.

1.) Data Acquisition is the first technical step in the data value chain. This includes gathering data from all key sources, including EHRs, satisfaction surveys, scheduling software, billing companies, and others.
This stage is rife with challenges, particularly for departments staffed by providers not employed by the health system. Special approvals and security clearances must first be agreed to by IT. This is where it is essential to select a data partner with prebuilt data-extract scripts, expertise in each EHR, and an automated process that requires little IT effort of computing resources.
Early considerations in acquiring data include setting up a secure data exchange process (i.e., SFTP) and discovering what structured data is available in EHR data tables and what data is only available in text. In addition, methods consistent with an HCO’s file formats and interfaces are established. These may include straightforward flat-file data pulls, HL7, and FHIR, as well as unstructured text.
In some cases, necessary data is not accessible in a usable format, especially in the case of unstructured text. For example, sometimes review of systems (ROS) observation data, physician attestations, and medical decision-making are not easily decipherable when unstructured text is taken from clinical notes. If it’s not possible to acquire usable unstructured text, a plan must be established to acquire structured data to serve as a potential surrogate.
In addition, automated quality assurance (QA) processes and procedures with medical specialty specific algorithms are required to catch data errors early in the data value chain to ensure data quality, integrity, and consistency. When errors are identified, remediation processes and procedures are triggered, engaging staff based on role and responsibility.
- Data fields missing
- Files not received when expected
- Invalid Data
- Hash totals or data values that fall outside a deviation parameter
Without a comprehensive data QA process in place, the data will not be trusted and the analytics will be of limited value.

2.) Data Cleaning is the process of identifying and clearing away or correcting inaccurate data before combining it with data from other sources.
Before any data is cleaned, it’s essential to gain an understanding of how operational processes vary between locations and how these differences may produce information that would otherwise be considered bad data upon first look. Identifying, assessing, and mitigating anomalies requires domain expertise.
After this type of assessment is complete, an inventory of site variations is created, as well as a data-cleaning code to standardize operational and clinical events. This will allow for intersite comparative analyses.
Common anomalies include what nomenclature is used and what operational processes are followed to tag patients as LWBS, AMA, or elopements. Other examples include how each site documents patient movement to ED observation, or the steps it follows to transfer a patient out of the ED.
These same anomalies are also considered when determining what is actually bad data when calculating turnaround time (TAT) metrics, and in reconciling duplicate data. For example, if a physician sees a patient during triage before the patient is put in a bed, the patient-in-bed-to-seen-by-doc time will be negative. If metrics are to be trusted, data must be cleaned to reflect how it is collected, local processes, and agreed-upon business rules.

3. ) Data Harmonization goes hand in hand with data cleaning. It refers to the process of integrating data in a way that enables it to be mined, studied, and analyzed. It creates a “single source of truth” by taking data from disparate sources and creating “gold standards.” Data elements and variables are identified, cleansed, and processed to create a data store that is unambiguous in how data is defined. It goes beyond simple interoperability (the focus of current legislation) to provide the data foundation needed to successfully plan and execute value-based care models.
Here, for example, we set up rules to reconcile differences in physician attribution, and for deciding how physicians and locations are compared. As the steps begin to merge, data harmonization leverages the work completed during the acquisition and cleaning stages.
What is learned from these two stages creates an understanding of why comparative metrics must be generated from transaction details or event logs instead of pre-aggregated hospital metrics that do not reflect how the ED and other areas really work. After completing the data harmonization stage, data extracts may need to be modified to reflect what has been learned.

4. ) Data Augmentation can help reduce the manual intervention required to develop meaningful information and insight into business data. Combining data elements and creating virtual fields facilitates rapid segmentation and virtually any type of multivariate analysis. Purposing this data and making it specific to the environment in which it will operate is critical.
For instance, adding the way a department is organized or the way it assigns practitioners can lead to relevant insights. Data augmentation makes a huge difference in specific areas, including:
- Creating clinical flags at the patient encounter level that group data by any dimension. This type of augmentation makes it easy to answer unanticipated questions without special programming.
- Adding criteria to encounters to determine which visits to include or exclude for MIPS measures. For example, for pediatric patients to be included for MIPS 66, we must determine which tests were performed. Without data augmentation, it is difficult to answer complex questions, such as, “What percentage of children diagnosed with pharyngitis had both an antibiotic ordered and a Group A strep test administered?”.
- Grouping ICD codes by diagnostic subgroups in order to perform meaningful analysis across more than 139,000 ICD10 procedure and diagnostic codes to identify patterns, trends, and correlations.
- Building rules to govern patient-provider attribution, grouping of medications (i.e., types of opioids with alternatives for managing pain), and for the way an ED is organized by areas such as mental health, trauma, pediatrics, and fast track

5.) Data Curation is a means of managing data integrated from diverse sources, making it more useful over time by incorporating historical trends, identifying new questions, and organically adding new data. Ideally, in addition to having curated data at the health system level, the data is aggregated across hundreds of sites and millions of visits to establish new knowledge and benchmarks.
Curated, anonymized data also can be combined across organizations for research and machine learning to train algorithms to generate new insights.
By directly embedding feedback, new questions, and benchmarks across a comprehensive set of interdependent metrics, data curation is organic, relevance stays current, and value grows. Data is structured for easy exploration by any dimension or measure across domains, from the highest to the most atomic level. This approach focuses the pace of innovation because a curated data set naturally expands to answer new questions.
Here’s one example of how curated data can be used to train and develop algorithms to improve patient flow:
- First, historical data on patient arrivals and acuity mix, provider productivity, and turnaround times is used to develop algorithms to load-balance and equitably assign patients. The goal here is to shorten ALOS, reduce LWBS, and even reduce physician burnout.
- Now take that one step further and provide data to formulate a crowding index in five-minute increments to feed the patient-assignment algorithm. Eliminating bottlenecks creates the opportunity to drive even greater value.
- Curated datasets and answered questions can be fed back, creating even richer datasets and algorithms. One current example is identifying the right staffing mix of physicians and advanced practice providers (APPs). APPs are a less expensive resource, but this may be a false economy once ordering patterns, case mix, ALOS, outcomes, and other key performance indicators are considered.
The Data Maturity Model for Physician Specialty Practices
Since a solid data foundation is required to formulate, activate, and sustain transformational value-based care initiatives, we need a roadmap to understand the scope and path forward. The d2i data maturity model is useful in evaluating data maturity in logical phases.
At each phase, physician groups are able to manage their practices better and proactively engage in conversations and decisions that create value.
- In the first phase, data available to practices is based on data generated by professional fee billing, and the focus is on revenue cycle management and basic operational measures.
- The second phase requires greater sophistication to acquire, integrate, and curate data from multiple source systems. This allows for a broad set of operational and clinical performance measures, enabling EM to participate in many quality improvement initiatives strategic to the health system.
- In the third phase, data from sources not historically within the purview of EM are integrated to let EM partner with hospital medicine and other services across the entire unscheduled acute care episode, including the 30-day period following discharge.

Conclusion
A decline in inpatient revenue is the new reality, necessitating a new paradigm where more revenue comes from ambulatory services and telemedicine, where patients who may previously have been admitted are instead safely discharged and referred to in-network services, and where EM takes on greater responsibility for care transitions and reducing returns.
With the right data at its disposal, and with a commitment by physician leaders to the data value chain, EM can prove its value and ensure its role in transforming unscheduled acute care.
Physicians are positioned to lead us to a health system of patient-centered care that balances cost, access, and quality. For that to happen, they need trusted, relevant, and actionable data.
However, physician leaders simply don’t have the time or experience to build a data management infrastructure — as described in this paper — to support their requirements. Even though HCOs already devote considerable resources to producing and collecting data, many struggle to leverage it to improve performance because essential steps in the data value chain are skipped or not resourced appropriately.
For physician groups, it comes down to three options:
- Depending on their hospital partners to provide a solution
- Building their own data analytics’ capacity
- Buying a solution.
Outsourced analytics, where the data value chain process is an inherent part of the solution, is a great option. Outsourcing can ensure a faster time-to-value, access to deep experience in data acquisition and management, and medical specialty domain expertise.
Outsourcing analytics also makes financial sense. It’s hard — and expensive — to maintain a fully staffed analytics department, particularly when talented, qualified candidates are in short supply. A study published in The Journal of the American Medical Informatics Association (JAMIA) indicated that health care providers are competing with vendors and insurance companies for a limited pool of experienced data scientists to support their analytics needs.
Finally, outsourcing analytics allows for a near-immediate return on your investment. With demand driving up the price of qualified talent, it may make more sense to hire outside help with purpose-built, out-of-the-box solutions to meet your needs and budget.
About the Authors
Alan Eisman
d2i Executive Vice President, Business Development
Alan Eisman has more than 30 years of experience in enterprise software, including in ERP, CRM, performance management, analytics, data management, and health care information technology.
He has a deep passion for igniting and leading change, especially in health care, where there’s an urgent need to move from fragmented care to integrated, value-based care. Eisman has worked closely with many health systems, including Mount Sinai Health, Northwell Health, NYU Langone, and Saint Luke’s, advising them on a broad range of data and analytics initiatives targeted toward financial, operations, and clinical performance improvement.
Before joining d2i, he served as senior vice president of sales and marketing at HBI Solutions, a leader in health care machine learning and predictive analytics, and as executive director of health care industry for Information Builders, where he grew health and life sciences industry revenues by more than 500%. Eisman, who received his initial training in information systems at IBM, has led high-growth teams at six software companies, including both early-stage and large, global firms.
Jonathan Rothman
d2i Founder and Chief Solutions Officer
Jonathan Rothman has more than 27 years of hands-on experience developing operational and business intelligence (BI) solutions for health care companies, with 20 years dedicated to emergency medicine. Rothman’s diverse background in health care includes insurance underwriting, managed care contracting, physician and hospital billing, and IT system selection, implementation, and support. He has been published in numerous books and articles on health care BI and has won or been nominated for national awards in the area of BI innovation specific to emergency medicine. Rothman received a B.S. in finance and an MBA in risk management from Temple University.
Rothman was previously the director of data management for a $120 million diversified, multi-entity health care specialty provider, where he architected and managed its business intelligence solution.
About d2i
d2i’s cloud-based performance analytic application cleans, harmonizes, augments, and curates data from various sources, providing EM-actionable insights that lead to performance optimization.
The company provides vital services to many of the largest EDs in the U.S., spanning the clinical, quality, financial, and operational domains. Its performance analytics application embeds best practices determined by details from more than 25 million ED visits.
In addition to its performance analytics application, d2i offers analytics services, sharing its extensive industry expertise and organic knowledge to ensure that client partners continue to stay ahead of the curve by rapidly identifying and implementing opportunities for improvement.
Explore our health care performance analytics solutions at www.d2ihc.com.
Trusted by Your Peers
We transform data for dozens of leading hospitals and health care organizations across the U.S.
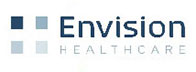
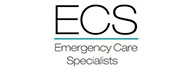

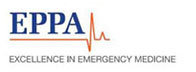

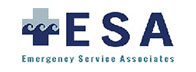
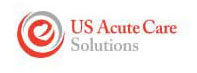
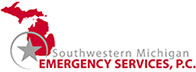


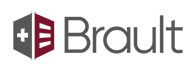
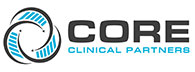
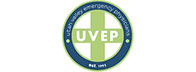
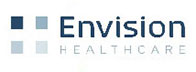
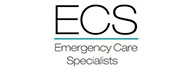

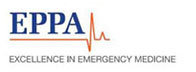

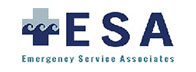
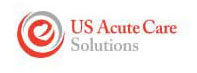
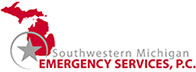


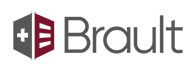
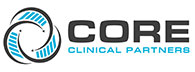
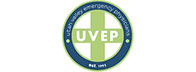